Gray areas include increasingly prevalent OCSEA-related behaviors such as self-generated explicit imagery and peer-to-peer offenses. These cases don’t have agreed-upon principles or guidelines, and the 昀椀eld has not yet decided how to handle them. For example, some companies choose to deprioritize self-generated explicit imagery when they enforce their content policies, while others treat it the same as third-party CSAM. The lack of standardized policies and enforcement approaches may lead to inconsistencies in the sharing and actioning of signals, limiting the effectiveness of the program. For example, signals related to edge cases and gray areas may not be uploaded to the Lantern Program database or they may go unaddressed even if they are uploaded by another company. Further, since the 昀椀eld has not yet established the best way to address gray areas, the use of the Lantern Program may increase the likelihood of human rights risk. For example, if companies share signals related to self-generated explicit imagery, there may be an increase in action taken against minors across platforms for distributing self-generated images, which may have adverse impacts on them, especially if they are criminalized. • Age determination, assurance, and veri昀椀cation are key challenges that may lead to increased risk for children. Age determination continues to be one of the biggest challenges in the OCSEA 昀椀eld. Determining the age of an individual in a sexual, explicit, or abusive content is challenging because adolescent children can be dif昀椀cult to distinguish from adults. This increases the likelihood that the industry underestimates the volume of child sexual abuse material because some images of children are classi昀椀ed as adults. Furthermore, it should be noted that some models currently used by tech companies have lower accuracy rates on children of color compared to white chil- dren, and children of color are more likely to be 昀氀agged as older than they actually are, which may 25 mean the image is not 昀氀agged as CSAM. Conversely, attempts to verify the age of users, particularly children, also come with risks, including potential adverse privacy impacts and inaccuracies based on race, gender, ethnicity, or culture. Currently, companies conduct age assurance by using biometric models or asking users to upload identi昀椀cation documents—both of which may lead to risks on privacy and data protection. Along with age determination, age assurance and veri昀椀cation are also major technical challenges for the 昀椀eld. Although the Lantern Program discourages participants from sharing personal information about children (e.g., in cases where a child is the offender), challenges around age assurance and veri昀椀cation may limit the ability of companies to effectively adhere to this rule. • Computer-generated CSAM brings new challenges to moderating OCSEA. Developments in diffusion models and generative AI technologies have enabled increased creation and distribution of synthetic CSAM, and it is proving increasingly challenging for the 昀椀eld to effectively distinguish between genuine or photorealistic computer-generated materials. Although computer-generated CSAM currently constitutes a small portion of online CSAM, its prevalence may increase rapidly. At the time of writing, tech companies do not have a clearly de昀椀ned approach to moderating computer-generated CSAM. One important factor when prioritizing action is determining whether a real-world victim is depicted in the materials or not. Companies may take different actions when moderating computer-generated CSAM if it does not involve real victims because their priority is to address real-world harm, though this is increasingly dif昀椀cult to distinguish. The proliferation of computer-generated CSAM may not only increase the scale and speed of OCSEA, but it may also lead to inconsistencies and challenges in content moderation and signal sharing. • Signal sharing may complicate the user appeals process and raise barriers to access remedy. 25 Stakeholder interview. BSR TECH COALITION HUMAN RIGHTS IMPACT ASSESSMENT 27
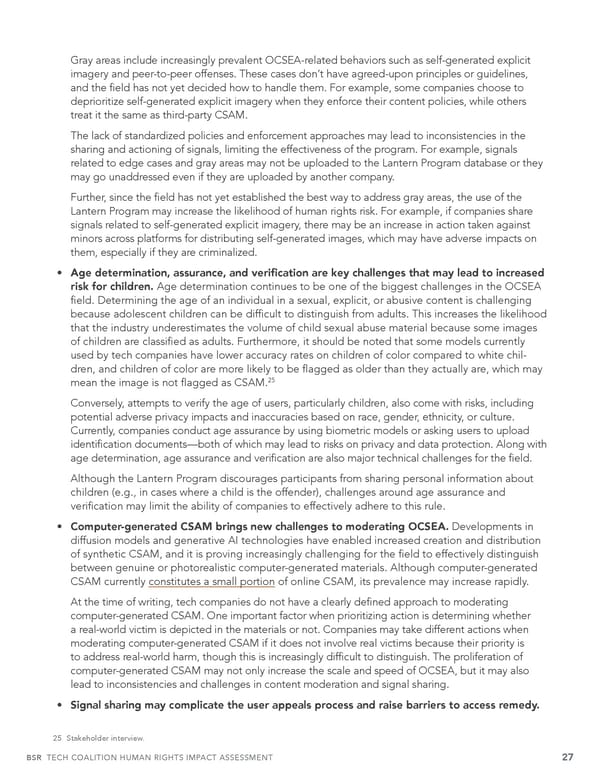